December 2021 Issue
Researcher Video Profiles
Toru Nakashika, Associate Professor Department of Computer and Network Engineering Graduate School of Informatics and Engineering University of Electro-Communications, Tokyo
Speech Signal Processing Based on Shallow Neural Networks
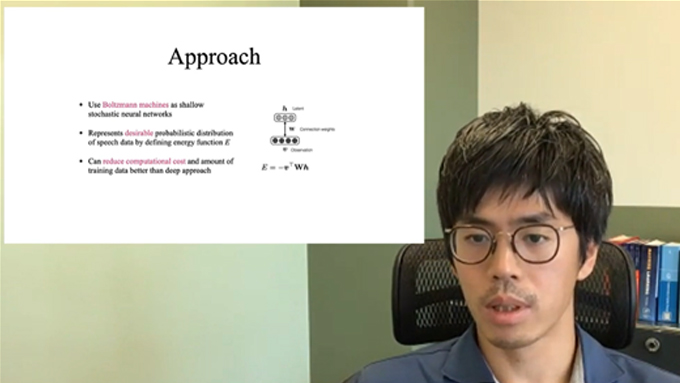
In this video feature Toru Nakashika describes his group’s research on speech signal processing using shallow neural networks.
It is widely known that deep learning (DL) is used in audio signal processing. In this approach, many studies use DL by increasing the number of layers of neural networks and parameters in the “dark cloud” to improve expressiveness and accuracy.
However, such models have problems such as high calculation costs and the need for huge amounts of data. Furthermore, DL is often called a black box, and it is difficult to interpret what is being done internally. Therefore, it is difficult to come up with ideas for improvements.
“The goal of my research is to produce the same level of accuracy in speech recognition and synthesis as in deep learning but by using interpretable and shallow models based on appropriately expressing the structure of speech data,” explains Nakashika. “That is by using wisdom instead of computational resources, we aim to reduce computational costs and achieve more practical speech recognition and speech synthesis.”
Nakashika and his colleagues use shallow models, including the Boltzmann machine model—an example of a shallow and interpretable model. The use of a Boltzmann machine enables the expression of an arbitrary probability distribution by freely designing so-called called energy functions, and audio data structures can be appropriately expressed using this model.
Since this Boltzmann machine is a shallow model, it has the advantage that both calculation costs and the amount of data required for learning reduced can be significantly reduced.
Some recent results obtained by Nakashika include voice identity conversion—a technology that processes voice and only converts a person's personality without changing the contents of the utterance. “I have proposed a model called the speaker-cluster-adaptive restricted Boltzmann machine,” says Nakashika. “This is an extension of the Boltzmann machine, and conversion is possible with only about one second of data.”
Nakashika has also proposed the so-called complex-valued restricted Boltzmann machine model that directly expresses complex numbers. Sound is often expressed in a complex spectrum, but since it is known that amplitude is better recognized by humans than phase, it is possible to omit the phase and only use the amplitude spectrum. “I think that it would be more expressive if there was a model that could directly express the phase, and the model that can directly express the complex spectrum of the voice is the complex-restricted Boltzmann machine mentioned earlier,” says Nakashika. “We showed that this makes it possible to synthesize speech with higher accuracy than the conventional VOice enCODER.”
Plans include the application of the Boltzmann machine to speech synthesis and voice quality conversion in other fields of speech signal processing, such as speech recognition and sound source separation. “I would like to encourage more promote more research on shallow neural networks.”