September 2021 Issue
Researcher Video Profiles
Shouhei Kidera, Associate Professor Department of Informatics Graduate School of Informatics and Engineering University of Electro-Communications, Tokyo
Radar-based human recognition for self-driving cars
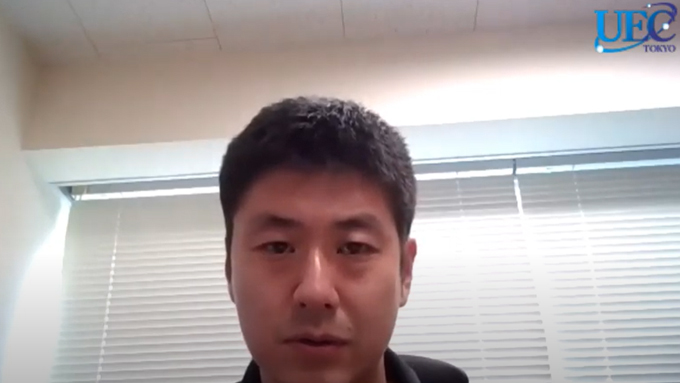
Shouhei Kidera and his group is focusing on recognizing pedestrians using millimeter wave radar—that enables sensing in darkness, fog, or rain—for self-driving cars. “We use millimeter wave radar because it is effective for recognizing pedestrians even in non-line-of-sight sensing scenarios by exploiting diffraction signals,” explains Kidera. “Most other radar based human recognition methods use micro-Doppler analysis. However, this approach needs higher Doppler velocity, temporal, and range resolution. Our approach is based on raw data machine learning, assuming Non-Line-of-Sight (NLOS) scenarios.”
Kitera and his colleagues use Frequency Modulated Continuous Wave Millimeter Wave (FMCW MMW) radar with 24 GHz center frequency, 700 MHz bandwidth, and horizontal and vertical beam widths of the radar of ± 45 degrees and ± 6.5 degrees, respectively.
In recent research they studied two different types of targets: a metallic cylinder, and a human with static motion and breathing [1].
In a Line of Sight scenario the team compared reflection responses from a human and metallic cylinder. “We obtained Gaussian distribution reflection spectra for a specific range,” says Kidera. “We confirmed the temporal variation of the response of a stationary human body in both amplitude and phase, which did not appear in cylinder target. These differences are promising for discriminating between human and metallic cylinders.”
Finally, the team investigated support vector machines (SVM) based machine learning using a variety of features with corresponding raw data (Details in the video).
Notably, the results on the recognition rate between human and metallic cylinder and SNR, and showed perfect recognition in LOS or partially NLOS situations. Also, in completely NLOS case, the recognition rate increased to 80 % by exploiting multiple temporal features, even in very lower SNR situations.