March 2021 Issue
Researcher Video Profiles
Yuichi Sei Associate Professor, Department of Informatics, Graduate School of Informatics and Engineering, University of Electro-Communications.
Web Internet of Things for analyzing data while protecting privacy
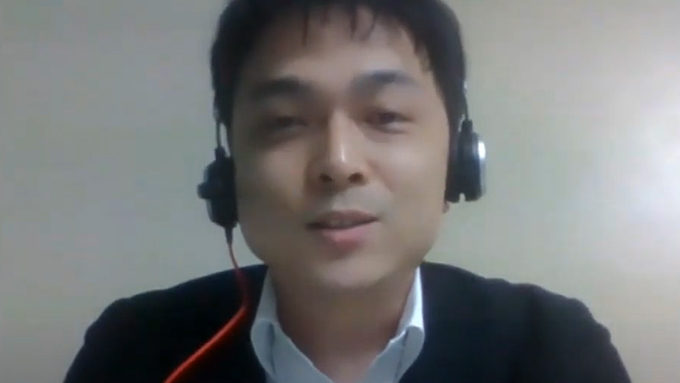
Associate Professor Yuichi Sei’s research is focused on analyzing data while protecting privacy. The background is to resolve privacy issues that arise due an increasing number of businesses using personal data that is collected from individuals, including voice, viewing, health, location, and train ticket gate data.
Notably, currently although such is data is protected it is also being provided to third parties after processing protection. So, there are increasing risks of leaks in privacy data.
For example, let's say a person uses social data such as Twitter and other social media, anonymously. This person is anonymous, but if we know that he often tweets about Chofu, conferences, research, and so on, we can assume that he is a university student or teacher near Chofu. If we know that he tweeted in August 2015, "What should I do about souvenirs for Helsinki soon. Then, we can deduce that he may be going to an academic conference in Helsinki around August 2015.
In addition, there is open data on "call for papers" on the web. The list of conferences is available, and papers submitted to conferences is also available. So it is easy to find a list of universities in Chofu. Also, universities generally publish lists of faculty members by job title and information on average income for each position.
By integrating this information, there is a risk that a third party, although anonymous, will be able to identify individuals, their occupations, and income levels. Furthermore, Tweets can be used to quickly make multiple guesses, which could be abused by third parties. Also recently, with advances in IoT technology, it is easy to obtain models trained for handling personal information, privacy-protected location data, health data, and other IoT data.
Although combining such "Web IoT Data" can be instructive, there are increasing risks from using inferred privacy information. Therefore, Sei and his colleagues are developing Web IoT that can understand and control privacy risks based on consideration of unexpected combinations, and performing machine learning and statistical analysis safely and with high accuracy, cross-sectional privacy protection, and data analysis.
They have proposed several privacy protection technologies, but the main target is to collect small amounts of perfect data without errors as personal data to be processed anonymously. For example, research has been conducted on obtaining accurate values without errors such as age, gender, and PCR test results.
“In the future, as IoT technology advances further, we will collect data that includes errors and defects observed in IoT and has many items, and analyze the data while protecting privacy for those data,” says Sei. An example of the research includes targeting data with inaccuracies such as age, gender, and the presence or absence of new coronavirus infection estimated from body temperature and perspiration volume produced by image recognition. They are developing technology for this purpose and collecting data for demonstration purposes.
It is essential that the method considers the errors and deficiencies in the data, so they have devised an algorithm for a new privacy protection index concept that protects real values that even the person himself does not know rather than protecting superficial ones. The measured values include errors and deficiencies.
Sei is also developing protection techniques that consider the combination of data, such as measuring the risk that data about the same person collected at different locations will be probabilistically identified as the same person when the data is published anonymously or processed anonymously.
Collecting actual data is one of the main problems to resolve in this research. So Sei and his colleagues are collecting experimental data by renting out two apartments in Chofu City, installing sensors and IoT home appliances, and asking participants to live in the apartments, and collected data, including personal data. In this way, they have also proposed methods and evaluating experiments based on actual data.
Fig 1 shows an example of some recent latest results assume a scenario where a person wants to know the histogram of the event participants' age from a camera image in a case where the resolution of the camera image is set to a low level for privacy reasons. In such a case, even if a machine learning model is applied, the accuracy of age prediction will be low for low resolution images.
Even if the prediction accuracy of individual machine learning models is low, Sei and his colleagues propose a technique to improve the accuracy of headcount counting. The black line shows the distribution of the data for the actual value shows the number of people by age group.
Drawing a line like this based on the prediction results for this age, the distribution will deviate slightly from the prediction, as shown in this baseline. However, their proposed method provides evidence that the statistical results are close to the actual distribution.
The second result is that of IoT data with many errors and deficiencies (Fig 2). Here when the analysis is carried out for multi-dimensional data based on such data, the amount of data with all the data is minimal, which makes it difficult to analyze.
Sei has also propose a method to reduce the error of the final statistical results by predicting the values corresponding to such missing data and thereby protecting privacy and has collected experimental data with various parameter settings and found that the proposed method has the smallest error.
In the future, Sei’s goal is to release the infrastructure we have developed as open-source to create and promote services that allow people to use data safely and freely.
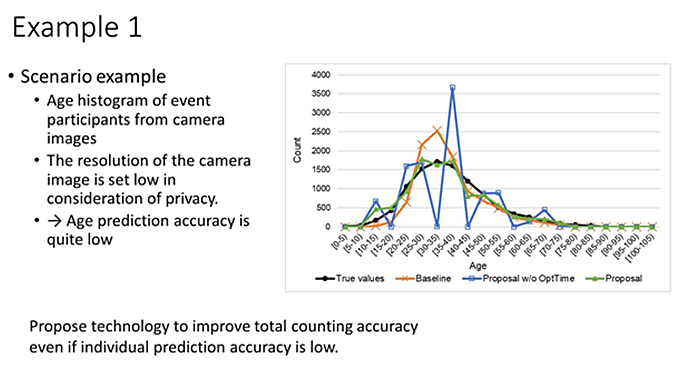
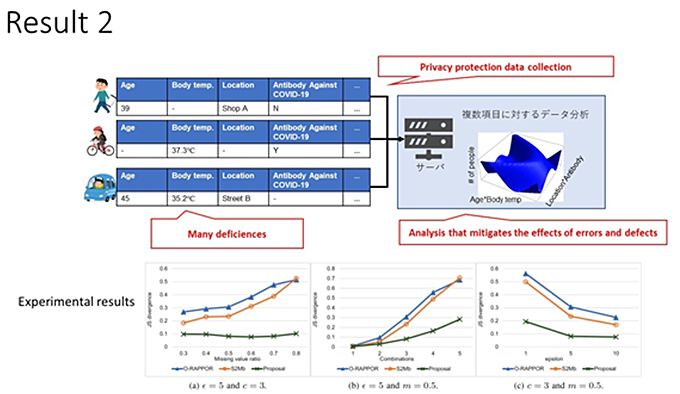