September 2018 Issue
Topics
Data science research on improving service design
Kazushi Okamoto, Assistant Professor, Department of Informatics, Graduate School of Informatics and Engineering, University of Electrocommunications, Tokyo.
Kazushi Okamoto conducts research on data science to improves services by discovering appropriate knowledge from data and applying it. His research is based on statistics, computer science, machine learning, computational intelligence, and knowledge about specific services. Okamoto is particularly interested in fundamental techniques and applications of collection and analysis of behavior logs such as purchase histories and access logs. He targets services that are provided in the real-world and cyberspace.
(1) The real-world case
In general, loan data for libraries is objective data that shows the facts of the usage of books borrowed. However, books, which are only used in the library and are not loaned, cannot be recorded and librarians do not have in depth knowledge about the usage of such books.
Kazushi Okamoto at UEC, Tokyo and colleagues at Chiba University and the National Museum of Japanese History, performed in-library book usage surveys using radio-frequency identification systems installed in bookshelves for two years [1].
They have analyzed the observed data in terms of the number of pickups and times books were used.
The analytical results suggest: (i) there frequency of pickups was three times greater than the loan of books; (ii) the peak usage time was two to four minutes; (iii) the peak pickup frequency and the peak usage time were different, and book usage styles differed depending on the season; (iv) most the of books were pickup only a few times.
It would be difficult to obtain these results from the loan statistics only, and research like this study is a new attempt to ascertain the facts about the usage of books.
(2) Cyberspace case
Kazushi Okamoto is studying collaborative filtering techniques that recommend related users or items with a user-item matrix (Figure 1). The user-item matrix consists of ratings by each user to each item, and the collaborative filtering techniques model the recommendation by word of mouth. Many e-commerce sites and on-line services implement collaborative filtering functions such as "people who bought the item, but also bought this item", but most of the mechanisms are a black box and it is difficult to know why these items are recommended.
Okamoto wants to develop a collaborative filtering method with an explanation function.
He is developing a model-based collaborative method using linear-multiple regression that constructs a recommendation model with training examples and calculates candidates using the model. The method suggests to users and system operators what users or items affect to the recommendation according to users' or items' weights within the model.
Currently, Okamoto is evaluating the recommendation accuracy, and in the future, he will develop a weights presentation method to users and verify its effectiveness.
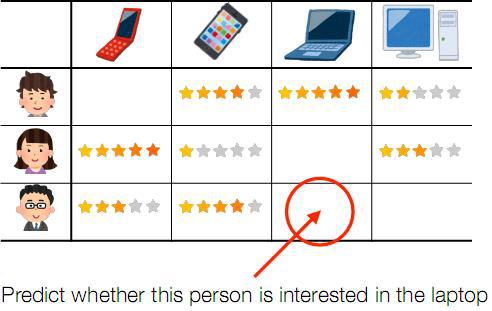
Reference
- [1] Kazushi Okamoto, Rie Marumo, Haruka Sano: In-Library Book Usage Survey by using Radio-Frequency Identification System Installed Bookshelves, Journal of Japan Society of Information and Knowledge, 27(3), 227-244, 2017. (in Japanese)