December 2019 Issue
Research Highlights
Inspecting dust on surfaces in product manufacturing: Effect of dirt on inspection surfaces on the accuracy of visual inspection
To supply high-quality products to the market, visual inspection by human senses is conducted in many manufacturing industries. It is generally recommended that visual inspection for a high-quality product be performed in a clean room.
However, in situations where the production process becomes more complex and more outsourcing in recent years, visual inspection is not often performed in a clean room because of constraints of existing equipment and economic problems.
Now, Ryosuke Nakajima at UEC Tokyo and colleagues at Aoyama Gakuin University have utilized peripheral vision to clarify experimentally the relationship between dirt levels of inspection surfaces and defect detection in visual inspection.
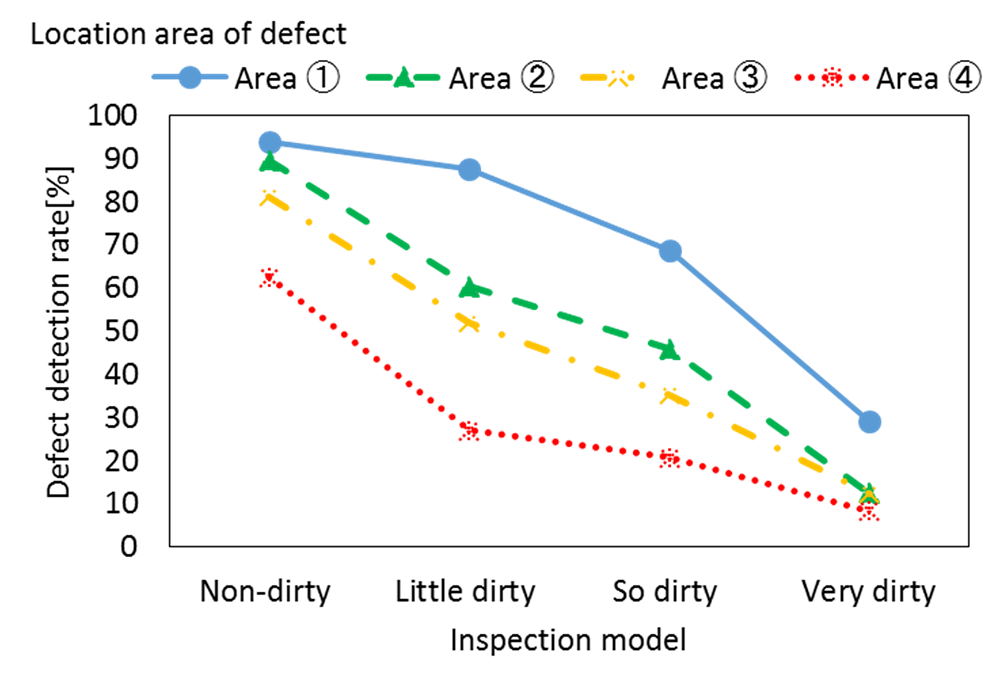
The experiment was a three step processes: analyze images of inspection surfaces in an actual factory and then create an inspection model for dirt density based on the pixel values of the images; design experiment using dirt levels of inspection surfaces, defect locations, and defect characteristics as experimental factors; examine the effects of these factors on the defect detection accuracy with 12 subjects.
A sudden reduction in the defect detection rate was observed as the inspection surface became dirtier.
The research shows the importance of a clean inspection surface for a highly accurate visual inspection process, and it is important to take measures such as making the production process in a clean room and cleaning an inspection surface before the visual inspection process.