September 2018 Issue
Research Highlights
Isotropization of Quaternion-Neural-Network-Based PolSAR Adaptive Land Classification in Poincare-Sphere Parameter Space
Quaternion neural networks (QNNs) achieve high accuracy in polarimetric synthetic aperture radar classification by working in Poincare-sphere-parameter space.
However, there are still anisotropic factors that lead to a classification capability degraded from its ideal performance.
Now, Fang Shang at the University of Electro-Communications, Tokyo and colleagues at the University of Tokyo, report an isotropic activation function for the QNN to improve the classification ability.
Fig.1(a) below shows how the conventional activation function maps input 3-D vectors, where vectors with lengths of two are taken as test samples. The input vectors distributed on a sphere are mapped to a cubic space with a side length of two and centered at the origin of the coordinate system. It means that the effect of the mapping process on a vector, i.e. the changes of length and direction, depends on the vector's spatial position. That is, the conventional activation function is anisotropic. Fig. 1(b) shows the situation of the proposed isotropic activation function that compresses the norm of a vector independent of its direction.
Fig.2 shows the results of classification experiments for ALOS-2 PALSAR2 data of Tomakomai area, Japan. The results indicate a great improvement in the classification ability of the isotropic QNN in comparison with the conventional one. The figures of the results were selected as the cover page of the issue of the IEEE journal where these results were published, (Fig.3).
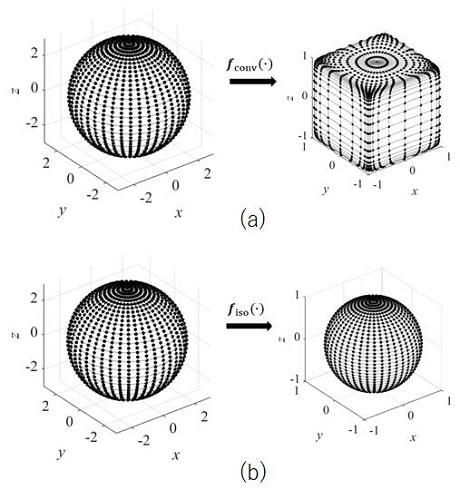
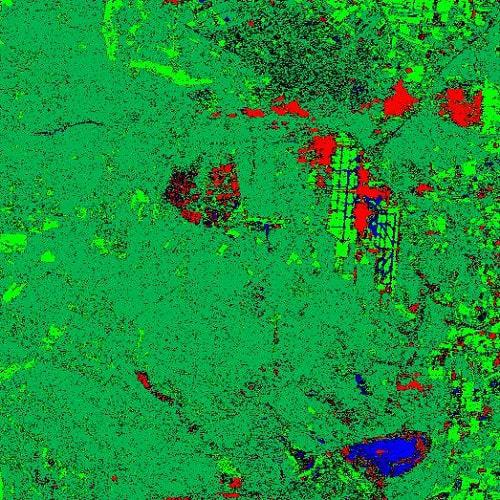
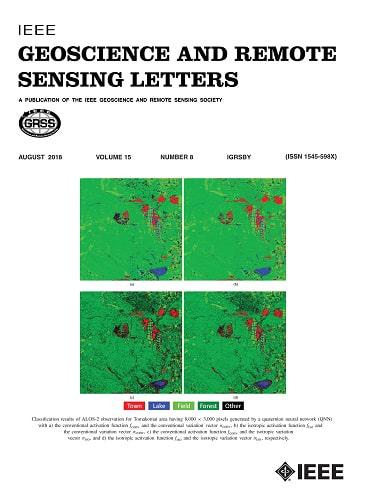