Video ProfilesInnovation
January 2024 Issue
AINet: AI for Innovative Wireless Networking
Katsuya Suto
Associate Professor, Graduate School of Informatics and Engineering
Associate Professor Katsuya Suto and his group is dedicated to revolutionizing wireless communications and networking through the integration of AI technologies.
Suto is focused on the integration of joint source and channel coding using deep neural networks. In current wireless communication systems like 4G and 5G, source coding caters to user services, but communication quality suffers due to non-optimized channel coding, leading to issues like block noise in video transmissions.
In contrast, the communication system being developed by Suto tailors both source and channel coding for user services, mitigating block noise in video transmissions. “We believe this system holds potential for future applications, such as object detection for autonomous vehicles,” says Suto.
In contrast, the communication system being developed by Suto tailors both source and channel coding for user services, mitigating block noise in video transmissions. “We believe this system holds potential for future applications, such as object detection for autonomous vehicles,” says Suto.
Another application of Suto’s communication system is integration with Google Maps. In the video, the mobile terminal estimates the radio map of user mobility from Google Maps, training the communication system accordingly. This ensures quality of service even in challenging wireless environments, offering stable video transmission during 5G outages.
In another application, Suto is addressing the high cost of radio map construction, by developing a low-cost solution using AI. By utilizing a 3D city model from open datasets like PLATEAU, Suto’s approach significantly reduces costs. Furthermore, the new system analyzes the radio path propagation process by the construction of a radio propagation graph. Implemented with shallow feedforward neural networks, this approach achieves twenty times faster computation than traditional ray tracing.
“Feel free to reach out for more information about our activities.”
References and further information
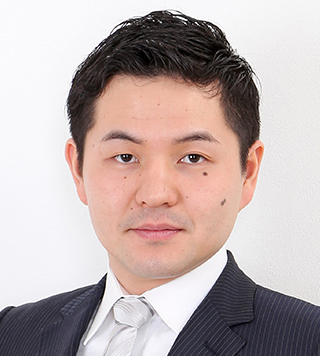
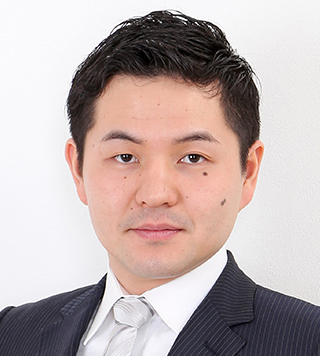
1. “Feel free to reach out for more information about our activities.”
DOI:10.1109/CCNC51644.2023.10059990
2. Katsuya Suto, Shinsuke Bannai, Koya Sato, Kei Inage, Koichi Adachi, and Takeo Fujii, "Image-Driven Spatial Interpolation with Deep Learning for Radio Map Construction," IEEE Wireless Communications Letters, vol. 10, no. 6, pp. 1222-1226, June 2021.
DOI:
10.1109/LWC.2021.3062666
3. Tiago Koketsu Rodrigues, Katsuya Suto, Hiroki Nishiyama, Jiajia Liu, and Nei Kato, "Machine Learning meets Computation and Communication Control in Evolving Edge and Cloud," IEEE Communications Surveys and Tutorials, vol. 22, no. 1, pp. 38-67, first quarter 2020.
DOI:
10.1109/COMST.2019.2943405