Research HighlightsInnovation
January 2025 Issue
Face recognitionVoice coming into the picture
During the COVID-19 pandemic, requiring people to wear facemasks in public places and shops was an important measure taken by governments all over the world. The requirement is no longer in place, but in Japan and elsewhere, many people still choose to wear a facemask as a precaution, and it is expected that facemasks will remain commonplace. This poses a problem to security and identification systems, as it is difficult to correctly automatically identify a person whose half face is covered by a mask. To address this situation, computational methods capable of ‘inpainting’ masked face images have been developed, with some degree of success. Now, Yuichi Sei and colleagues from The University of Electro-Communications, Tokyo, have created a method that not only uses visual information, but also audio — the person’s voice — to reconstruct a masked face. The approach enables face shape restoration with improved quality.
The researchers were led by the notion that a relationship exists between a person’s face shape and the person’s voice. Indeed, facial muscles and the shape of the nasal cavity, for example, contribute to how a voice sounds. In addition, a person’s age, gender and ethnicity have an impact on intonation. Importantly, relevant scenarios for which both visual and audio information are available are conceivable, for example audiovisual recordings of a crime scene in which the criminal wears a mask.
Partly based on an earlier, machine-learning method for face completion, the approach of Sei and colleagues required a data set for training and testing the method. To this end, the researchers used a database with face (pictures) and voice (audio recordings) information of 1,225 people. It is worth noting that much more than one picture and one audio recording were available per person (in total, almost 300,000 picture and voice files were used). Masked face images were created from unmasked images by erasing out the areas that would be covered by a randomly chosen mask type.
Comparisons between the unmasked images and the inpainted images confirmed the high quality of the achieved face shape restoration. Situations in which the reconstruction worked particularly well were faces with sharp contours, and faces with high nasal heads.
It should be noted that the data used as input was in fact a combination of two different datasets: one for the faces, and one for the voices. This means that a face image did not necessarily correspond to the actual face during the utterance of the voice. Moreover, there may have been discrepancies due to a person’s face shape and voice quality changing with time. Nevertheless, the quality of the results obtained with the approach of Sei and colleagues was better than that for earlier methods. Quoting the scientists: “[Our] experimental results show that the proposed method improves the quality both qualitatively and quantitatively compared to the method without voice.”
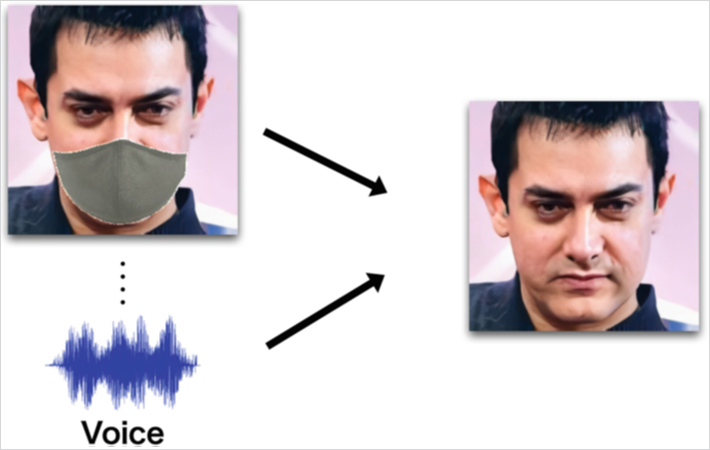
[Fig. 1 from the paper]
A person’s masked face is reconstructed by including audio of the person’s voice.
References
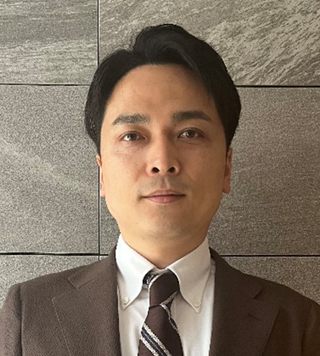
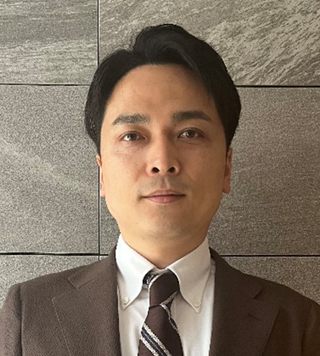
Tetsumaru Akatsuka , Ryohei Orihara , Yuichi Sei ,Yasuyuki Tahara , and Akihiko Ohsuga, Estimation of Unmasked Face Images Based on Voice and 3DMM, In: Liu, T., Webb, G., Yue, L., Wang, D. (eds) AI 2023: Advances in Artificial Intelligence. AI 2023. Lecture Notes in Computer Science (LNCS), volume 14471. Springer, Singapore.
URL:
https://doi.org/10.1007/978-981-99-8388-9_20
DOI:
10.1007/978-981-99-8388-9_20
Sei Lab Home Page
http://www.sei.lab.uec.ac.jp/~sei/en/